Google DeepMind: Subtle Adversarial Image Manipulation Influences Both AI Model and Human Perception
Recent DeepMind research reveals that subtle adversarial image manipulations, originally designed to deceive AI models, also subtly influence human perception. This discovery underscores similarities and distinctions in human and machine vision, emphasizing the need for further research in AI safety and security.
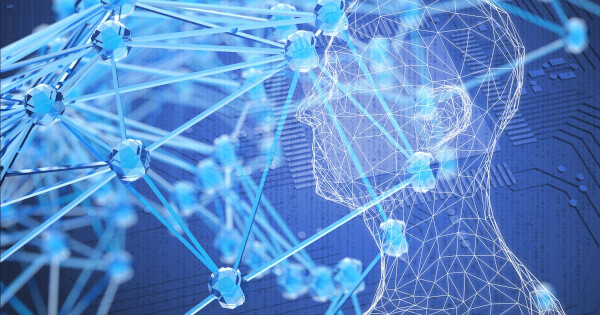
Recent research by Google DeepMind has revealed a surprising intersection between human and machine vision, particularly in their susceptibility to adversarial images. Adversarial images are digital images subtly altered to deceive AI models, making them misclassify the image contents. For example, a vase could be misclassified as a cat by the AI.
The study published in "Nature Communications" titled "Subtle adversarial image manipulations influence both human and machine perception" conducted a series of experiments to investigate the impact of adversarial images on human perception. These experiments found that while adversarial perturbations significantly mislead machines, they can also subtly influence human perception. Notably, the effect on human decision-making was consistent with the misclassifications made by AI models, albeit not as pronounced. This discovery underlines the nuanced relationship between human and machine vision, showing that both can be influenced by minor perturbations in an image, even if the perturbation magnitudes are small and the viewing times are extended.
DeepMind's research also explored the properties of artificial neural network (ANN) models that contribute to this susceptibility. They studied two ANN architectures: convolutional networks and self-attention architectures. Convolutional networks, inspired by the primate visual system, apply static local filters across the visual field, building a hierarchical representation. In contrast, self-attention architectures, originally designed for natural language processing, use nonlocal operations for global communication across the entire image space, showing a stronger bias toward shape features than texture features. These models were found to be aligned with human perception in terms of bias direction. Interestingly, adversarial images generated by self-attention models were more likely to influence human choices than those generated by convolutional models, indicating a closer alignment with human visual perception.
The research highlights the critical role of subtle, higher-order statistics of natural images in aligning human and machine perception. Both humans and machines are sensitive to these subtle statistical structures in images. This alignment suggests a potential avenue for improving ANN models, making them more robust and less susceptible to adversarial attacks. It also points to the need for further research into the shared sensitivities between human and machine vision, which could provide valuable insights into the mechanisms and theories of the human visual system. The discovery of these shared sensitivities between humans and machines has significant implications for AI safety and security, suggesting that adversarial perturbations could be exploited in real-world settings to subtly bias human perception and decision-making.
In summary, this research presents a significant step forward in understanding the intricate relationship between human and machine perception, highlighting the similarities and differences in their responses to adversarial images. It underscores the need for ongoing research in AI safety and security, particularly in understanding and mitigating the potential impacts of adversarial attacks on both AI systems and human perception.
Image source: Shutterstock